Executive Summary
- Understanding Customer Service Analytics—This involves collecting and analyzing customer data from multiple touchpoints to enhance customer experience by identifying behavior patterns and areas for improvement.
- Benefits of Customer Service Analytics—Businesses can identify trends, improve customer satisfaction, optimize resources, and reduce churn by leveraging insights from analytics.
- Implementation Strategies—Effective customer service analytics require identifying key metrics, collecting data from diverse touchpoints, using analytics tools, and acting on insights for continuous improvement.
- Role of AI and Advanced Analytics—Tools like LiveX AI offer AI-powered solutions that integrate with existing platforms to provide real-time insights, improve customer engagement, and reduce churn.
In today's competitive market, implementing customer service analytics is essential for delivering exceptional customer experiences. Leveraging AI-driven solutions can help businesses gain a deep understanding of customer needs, optimize interactions, and drive long-term success.
What is Customer Service Analytics?
Customer service analytics is the practice of collecting, measuring, and analyzing customer data from various touchpoints to gain actionable insights and improve the overall customer experience. It involves capturing data from customer interactions such as support tickets, live chats, social media mentions, surveys, and purchase history. The primary goal of customer service analytics is to understand customer needs, preferences, and behavior patterns to identify areas for improvement and deliver exceptional customer service.
By leveraging customer service analytics, businesses can uncover valuable insights that help them make data-driven decisions. For instance, analyzing support ticket data can reveal common issues customers face, enabling companies to proactively address them and reduce ticket volume. Similarly, monitoring customer sentiment through social media and survey feedback can help identify customer pain points and prioritize improvements.
The benefits of implementing customer service analytics are manifold. It enables businesses to:
- Identify trends and patterns: Customer service analytics helps uncover recurring issues, popular features, and customer preferences, allowing companies to stay ahead of the curve.
- Improve customer satisfaction: By understanding customer needs and addressing their concerns proactively, businesses can enhance customer satisfaction and build long-term loyalty.
- Optimize resource allocation: Analytics can help identify peak hours, common issues, and agent performance, enabling efficient resource allocation and reducing response times.
- Reduce churn: By identifying at-risk customers and addressing their concerns proactively, businesses can minimize churn and improve customer retention.
To implement customer service analytics effectively, businesses need to:
- Identify key metrics and KPIs that align with their customer service goals
- Collect data from various touchpoints such as support tickets, live chats, and surveys
- Use analytics tools to process and analyze the data
- Create visualizations and dashboards to make insights easily accessible
- Regularly review and act upon the insights to drive continuous improvement
Tools like LiveX AI Agents can help businesses streamline their customer service analytics process. LiveX AI offers AI-powered solutions that integrate with existing customer service platforms, providing real-time insights and enabling proactive customer engagement. By leveraging LiveX AI's AI Chat, AI Search, and AI ChurnControl, businesses can reduce churn, enhance customer retention, and improve trial conversions.
For example, Akool, an AI platform for personalized visual marketing and advertising, experienced a 26.4% reduction in customer churn within the first month and a remarkable 40x+ ROI after integrating LiveX AI ChurnControl. By identifying at-risk customers and proactively addressing their concerns, Akool was able to significantly improve customer retention and drive business growth.
In today's competitive landscape, customer service analytics is no longer a nice-to-have but a must-have for businesses looking to deliver exceptional customer experiences. By leveraging the power of data and AI-driven solutions like LiveX AI, companies can gain a deep understanding of their customers, identify improvement opportunities, and drive long-term success.
How to Implement Customer Service Analytics
Implementing customer service analytics involves a systematic approach to data collection, analysis, and integration. The first step is to gather data from various customer touchpoints, such as:
- Support tickets: Capture data from customer inquiries, complaints, and feedback received through your ticketing system.
- Live chat: Monitor and analyze customer conversations with support agents to identify common issues and sentiment.
- Social media: Track mentions, comments, and messages across social media platforms to gauge customer sentiment and identify trends.
- Surveys: Collect feedback through post-interaction surveys, NPS surveys, and customer satisfaction surveys.
- Purchase history: Analyze customer purchase patterns, preferences, and behavior to identify upsell and cross-sell opportunities.
Once data is collected, employ advanced analytical tools and techniques to process and derive insights from the information. This may include:
- Text analytics: Use natural language processing (NLP) and sentiment analysis to extract insights from unstructured data like support tickets and social media mentions.
- Predictive analytics: Leverage machine learning algorithms to predict customer behavior, churn risk, and lifetime value.
- Data visualization: Create interactive dashboards and reports to make insights easily accessible and actionable for customer service teams.
To maximize the impact of customer service analytics, ensure seamless integration with your existing customer relationship management (CRM) system. This allows for a unified view of customer data and enables personalized interactions based on insights gained from analytics.
LiveX AI's AI-powered solutions integrate effortlessly with popular CRM platforms, empowering businesses to deliver exceptional customer experiences. For instance, LiveX AI's AI Chat uses natural language understanding to provide instant, personalized responses to customer inquiries, reducing response times and improving satisfaction. Jobright, a leading recruitment platform, saw a 30% reduction in support ticket volume after implementing LiveX AI's AI Chat.
The true value of customer service analytics lies in translating insights into actionable strategies. Some ways to leverage analytics for customer service improvement include:
- Proactive support: Identify common issues and proactively reach out to customers with solutions before they encounter problems.
- Personalized experiences: Use customer data to tailor interactions, offers, and recommendations based on individual preferences and behavior.
- Self-service optimization: Identify areas where customers struggle and optimize self-service resources like FAQs and knowledge bases to reduce support volume.
- Agent training: Use analytics to identify skill gaps and training opportunities for customer service agents, improving their ability to handle complex issues.
Implementing customer service analytics is an ongoing process that requires continuous monitoring, refinement, and adaptation. By regularly reviewing insights and making data-driven decisions, businesses can stay ahead of customer needs and deliver exceptional experiences that drive loyalty and growth.
What are the Key Components of Customer Analytics?
Customer analytics encompasses several key components that work together to provide a comprehensive understanding of customer behavior and service performance. These components include:
Descriptive Analytics
Descriptive analytics provides a summary of historical data, allowing businesses to understand what has happened in the past. This type of analytics focuses on aggregating and presenting data in a meaningful way, such as through visualizations and reports.
By analyzing past customer interactions, purchases, and feedback, descriptive analytics helps identify patterns and trends in customer behavior. This information is useful for understanding:
- Customer segments: Identifying distinct groups of customers based on demographics, preferences, or behavior.
- Service performance: Evaluating the efficiency and effectiveness of customer service channels and agents.
- Peak demand periods: Determining times when customer service volume is highest, allowing for better resource allocation.
Descriptive analytics forms the foundation for more advanced analytics techniques, providing a clear picture of past customer behavior and service performance.
Diagnostic Analytics
Diagnostic analytics takes descriptive analytics a step further by examining data relationships to identify the reasons behind past outcomes. This type of analytics helps answer the question, "Why did this happen?"
For example, if descriptive analytics reveals a spike in customer complaints, diagnostic analytics can help pinpoint the specific causes, such as:
- Product defects: Identifying issues with product quality or functionality that lead to customer dissatisfaction.
- Service delays: Determining bottlenecks in the customer service process that result in longer wait times or unresolved issues.
- Agent performance: Analyzing individual agent performance to identify areas for improvement or additional training.
By understanding the root causes of customer complaints or service delays, businesses can take targeted actions to address these issues and improve the overall customer experience.
Predictive Analytics
Predictive analytics uses historical data, machine learning algorithms, and statistical models to make predictions about future customer behavior and service needs. This type of analytics helps businesses anticipate customer actions and proactively address potential issues.
Some common applications of predictive analytics in customer service include:
- Churn prediction: Identifying customers who are likely to churn based on factors such as decreased engagement, negative sentiment, or service issues.
- Demand forecasting: Predicting customer service volume based on historical patterns, allowing for optimal staffing and resource allocation.
- Upsell and cross-sell opportunities: Identifying customers who are likely to purchase additional products or services based on their past behavior and preferences.
By leveraging predictive analytics, businesses can take a proactive approach to customer service, addressing potential issues before they arise and capitalizing on opportunities to enhance customer relationships.
Prescriptive Analytics
Prescriptive analytics takes predictive analytics a step further by not only predicting future outcomes but also providing recommendations for the best course of action. This type of analytics helps answer the question, "What should we do?"
In the context of customer service, prescriptive analytics can provide recommendations for:
- Personalized offers: Suggesting targeted promotions or discounts based on a customer's purchase history and preferences.
- Service channel optimization: Recommending the most effective service channel (e.g., phone, email, chat) for a given customer based on their past interactions and preferences.
- Agent assignment: Matching customers with the most suitable service agent based on factors such as skill set, experience, and customer personality.
By providing actionable recommendations, prescriptive analytics empowers businesses to make data-driven decisions that optimize the customer experience and drive business success.
LiveX AI's AI Search leverages prescriptive analytics to provide personalized search results and recommendations to customers. By analyzing customer data, such as search history, click behavior, and purchase patterns, AI Search suggests relevant products, articles, or support resources that best meet each customer's unique needs. This not only improves the customer experience but also drives increased conversion rates and revenue.
How to Use Predictive Analytics in Customer Service
Predictive analytics is a powerful tool for enhancing customer service by leveraging historical data patterns to forecast future customer behaviors, preferences, and needs. By implementing predictive analytics, businesses can proactively address potential issues, optimize resource allocation, and deliver personalized experiences that drive customer satisfaction and loyalty.
Here are four key ways to use predictive analytics in customer service:
- Forecasting customer behavior: Predictive analytics allows businesses to anticipate future customer actions based on past data patterns. By analyzing factors such as purchase history, website interactions, and customer service inquiries, businesses can identify trends and predict:
- Likely customer purchases
- Potential service issues
- Probable churn risks
Armed with this information, customer service teams can proactively reach out to customers with relevant offers, support, or retention strategies.
- Personalizing customer interactions: Predictive analytics enables businesses to tailor customer interactions based on individual preferences and needs. By analyzing customer data, such as:some text
- Demographics
- Purchase history
- Communication preferences
- Sentiment analysis

Businesses can create targeted offers, recommend relevant products, and deliver personalized service experiences that resonate with each customer.
- Optimizing resource allocation: Predictive analytics can help businesses forecast peak service times and optimize staff and resource allocation accordingly. By analyzing historical data on:some text
- Call volumes
- Chat inquiries
- Email requests
Businesses can predict when customer service demand will be highest and ensure adequate staffing to maintain service quality and minimize wait times.
- Managing churn risks: Predictive analytics plays a crucial role in identifying customers who are at risk of churning. By analyzing factors such as:
- Decreased engagement
- Negative sentiment
- Service issues
- Competitive offers
Businesses can proactively intervene with targeted retention strategies, such as personalized offers, proactive support, or loyalty rewards, to prevent customer churn and maintain long-term relationships.
How LiveX AI Enhances Customer Experience with Analytics
LiveX AI is at the forefront of leveraging customer analytics to transform customer interactions into exceptional experiences. With a suite of AI-driven solutions, LiveX AI empowers businesses to reduce churn, enhance customer retention, and improve trial conversions through personalized engagement.
Here are four key ways LiveX AI enhances customer experience with analytics:
- AI Integration: LiveX AI offers seamless integration with existing customer service platforms, allowing businesses to leverage AI-powered analytics without disrupting their current workflows. By integrating LiveX AI's tools, such as:some text
- AI Chat
- AI Search
- AI ChurnControl
Businesses can quickly and easily access advanced analytics capabilities to optimize customer interactions and drive business success.
- Real-Time Insights: LiveX AI provides businesses with real-time analytics and insights, empowering them to make data-driven decisions that optimize the customer experience. With LiveX AI's dashboards and reporting tools, businesses can:some text
- Monitor key performance indicators (KPIs) such as customer satisfaction, resolution times, and conversion rates.
- Identify trends and patterns in customer behavior and service performance.
- Measure the impact of service improvements and personalization efforts.
By leveraging real-time insights, businesses can continuously refine their customer service strategies and adapt to changing customer needs and preferences.
- Efficiency and Automation: LiveX AI's solutions automate repetitive tasks and streamline customer service processes, allowing businesses to focus on delivering high-quality, personalized experiences. For example:some text
- AI Chat can handle routine inquiries and support requests, freeing up human agents to focus on more complex issues.
- AI Search can quickly surface relevant information and resources, reducing the time customers spend searching for answers.
- AI ChurnControl can automatically address the concerns of at-risk customers, enabling targeted retention efforts.
By leveraging automation and efficiency gains, businesses can optimize their customer service operations, reduce costs, and improve overall customer satisfaction.
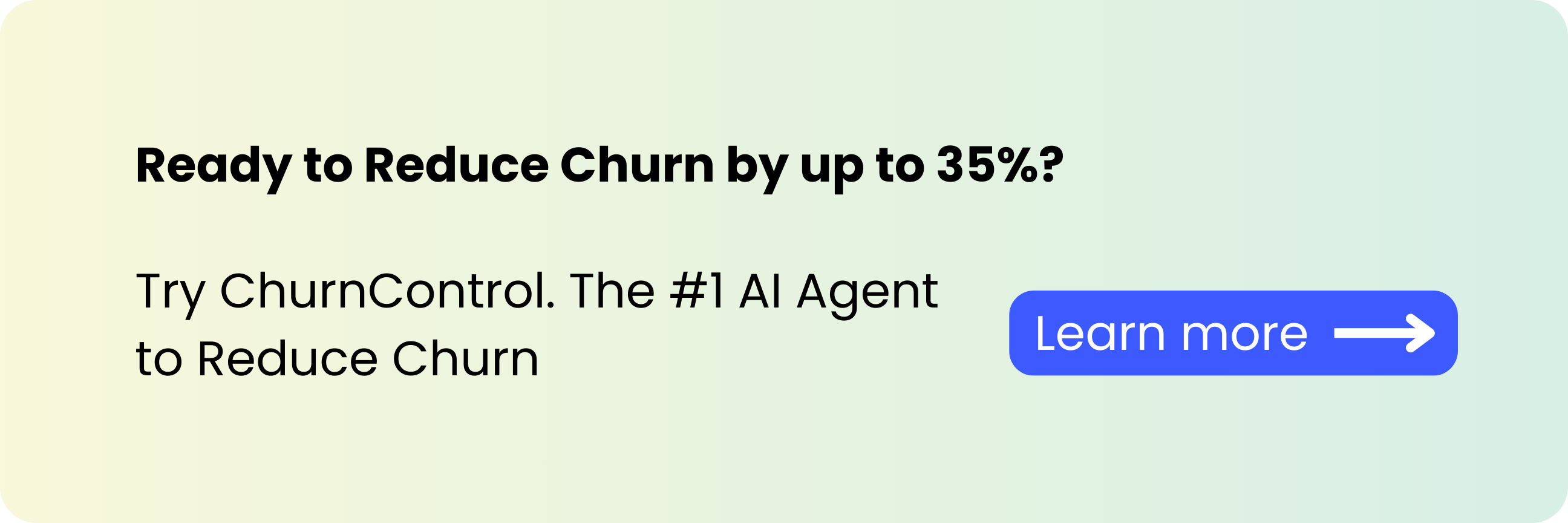